Genetic studies
A fully data-driven approach for genome-wide-association stuides (GWAS) with image-based phenotypes
Image-derived phenotypes - traits extracted from biological images - capture rich morphological information and understanding their genetic basis is crucial for elucidating developmental mechanisms and linking genetic variation to complex visual traits, relevant in many areas of biomedical, evolutionary, and forensic research and applications. However, there are key limitations in the current methodology, such as in the degree of which the large image complexity is captured with the phenotyping methods and how the genetic analysis methods deal with the underlying large genetic complexity. Moreover, needed multi-cohort studies are constrained by privacy regulations often prohibit sharing individual image data across institutions. Here, we present a robust, scalable, privacy-preserving analysis pipeline for unveiling the genetic basis of image-based complex traits, integrating (i) AI-based phenotyping for automatically extracting large numbers of endophenotypes; (ii) Combined-GWAS (C-GWAS) for identifying genetic variants underlying the numerous endophenotypes; (iii) federated learning for training AI-based phenotyping models across multiple cohorts without sharing individual images; and (iv) explainable AI for image-based visualization of the identified genetic effects.
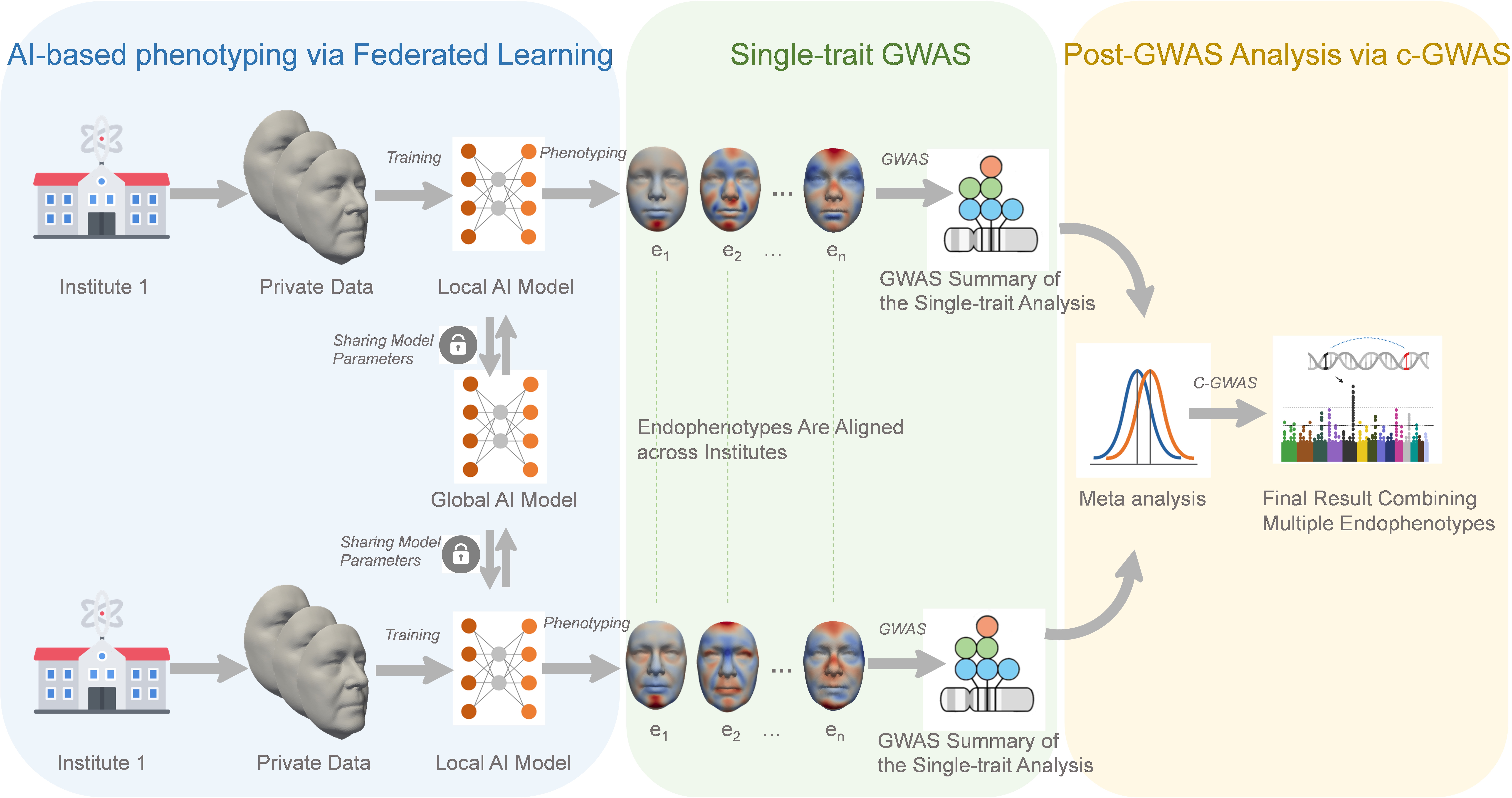
Figure below shows the Manhattan plot of our c-GWAS results, together with facial phenotypes associated with each leading SNP. In total of 43 genomic loci were identified, of which 12 have not been previously reported in existing studies.
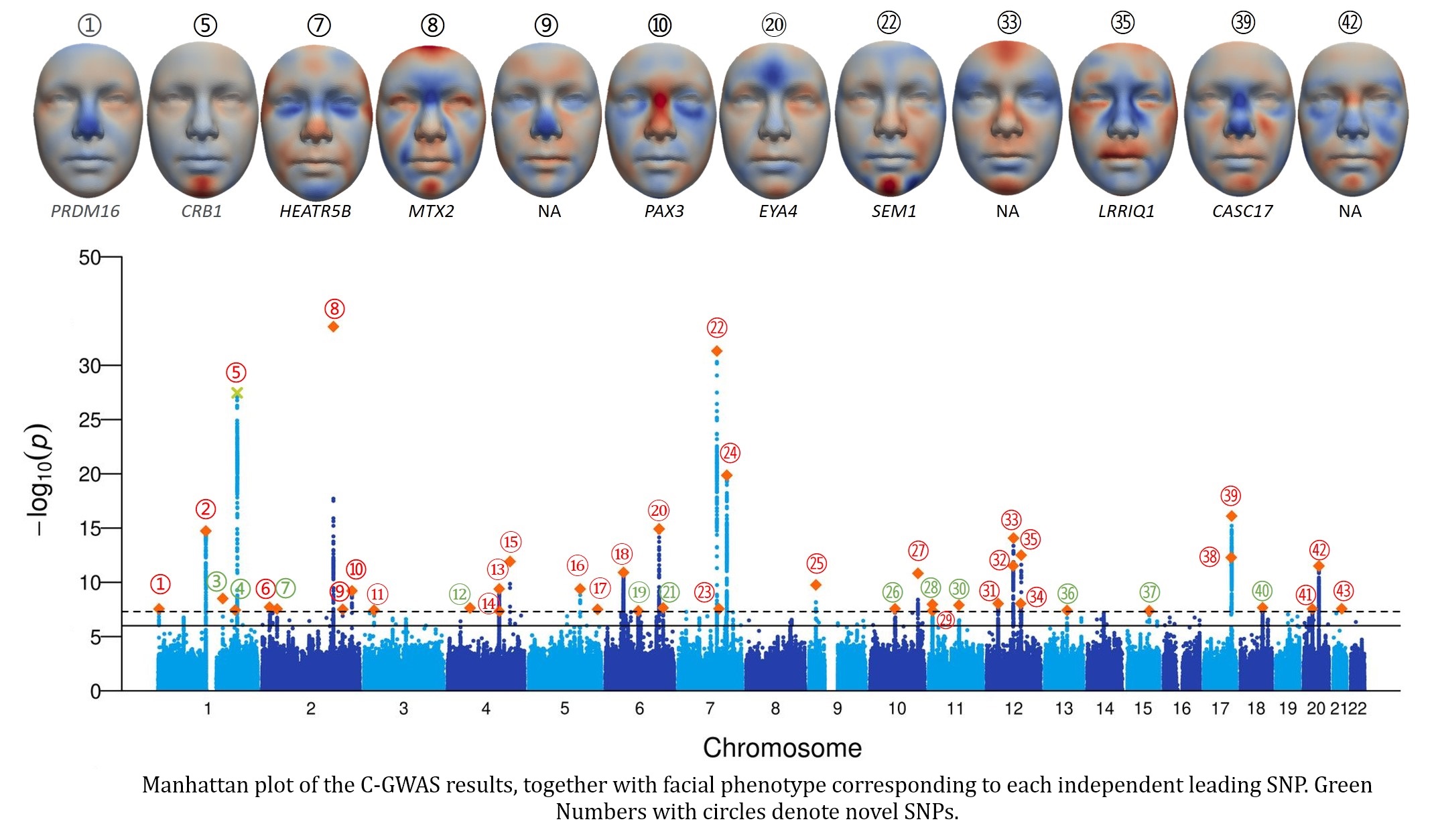