AI-based association analysis
We developed a novel AI tool to overcome the interpretability and confounding issues in deep-learning-based association analysis.
AI has greatly enhanced medical image analysis, yet its use in epidemiological imaging studies remains limited due to visualization challenges in non-linear models and confounder control. Addressing this, we introduce an AI method emphasizing semantic feature interpretation and resilience against multiple confounders. Our approachโs merits are tested in three scenarios: 1) extracting confounder-free features from a 2D synthetic dataset; 2) assessing the link between prenatal alcohol exposure and childrenโs facial shapes using 3D mesh data; 3) exploring the relationship between global cognition and brain images with a 3D MRI dataset.
The scripts for this project can be found in this Gitlab link.
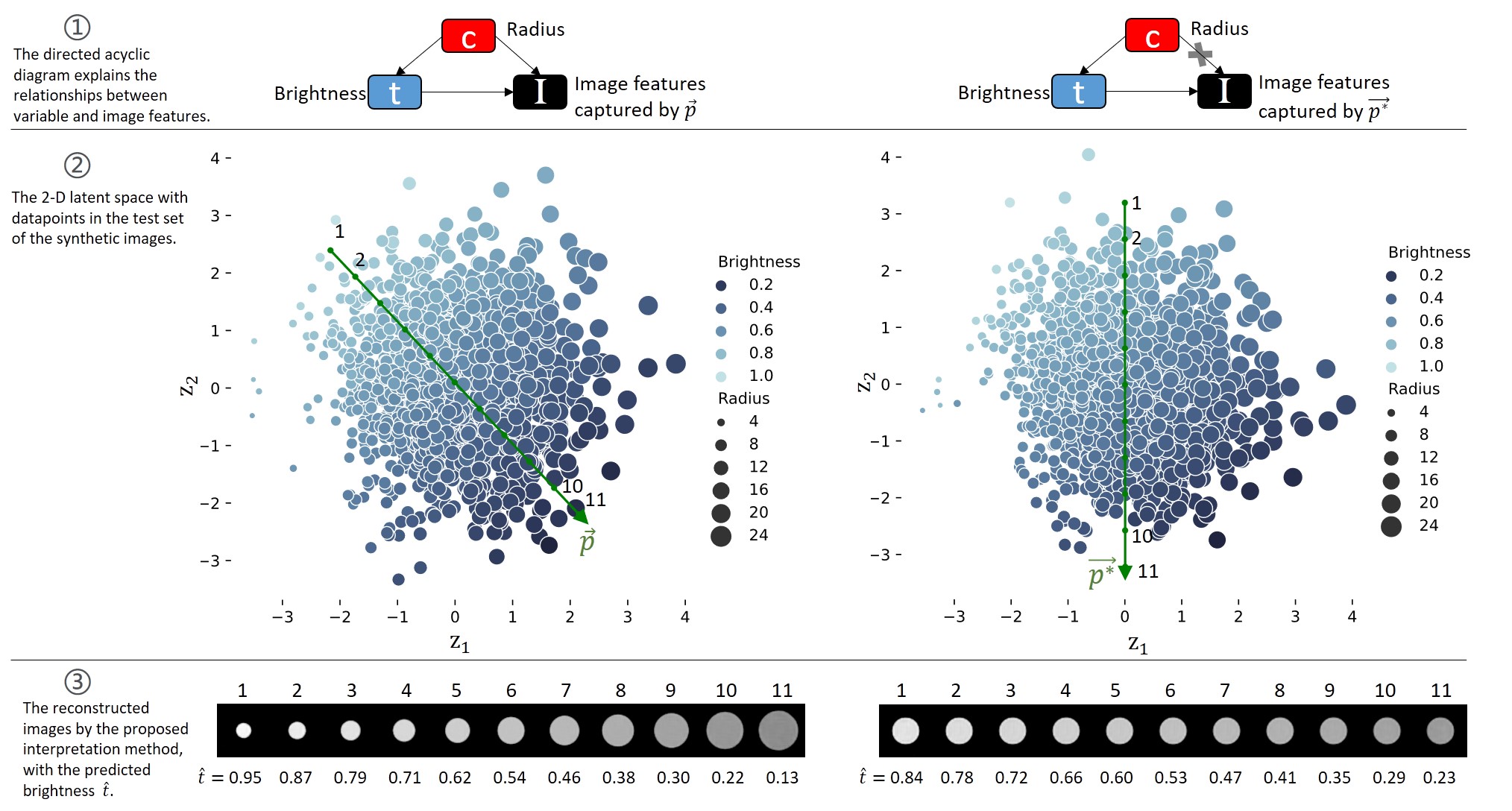
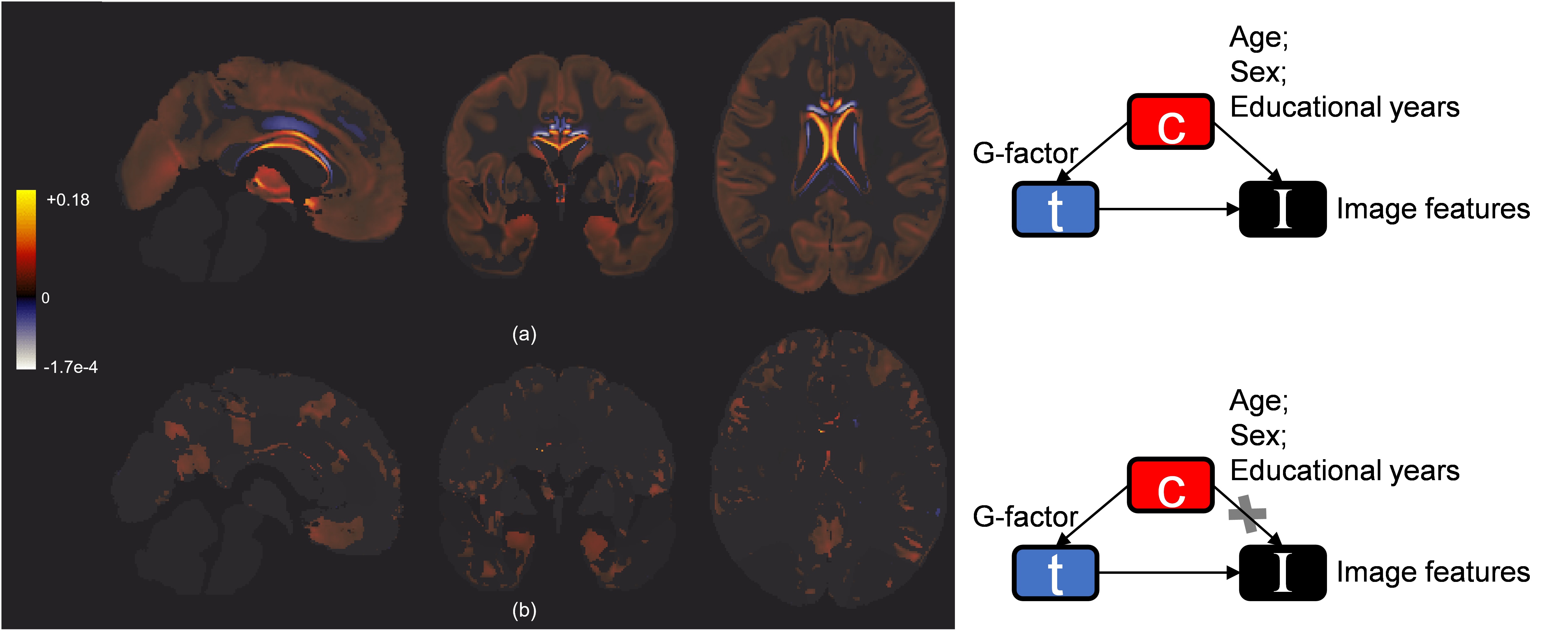
References
2023
- arXivAI-based association analysis for medical imaging using latent-space geometric confounder correctionarXiv, Nov 2023
2021
- miccai 2021Projection-Wise Disentangling for Fair and Interpretable Representation Learning. Application to 3D Facial Shape AnalysisLecture Notes in Computer Science, Sep 2021